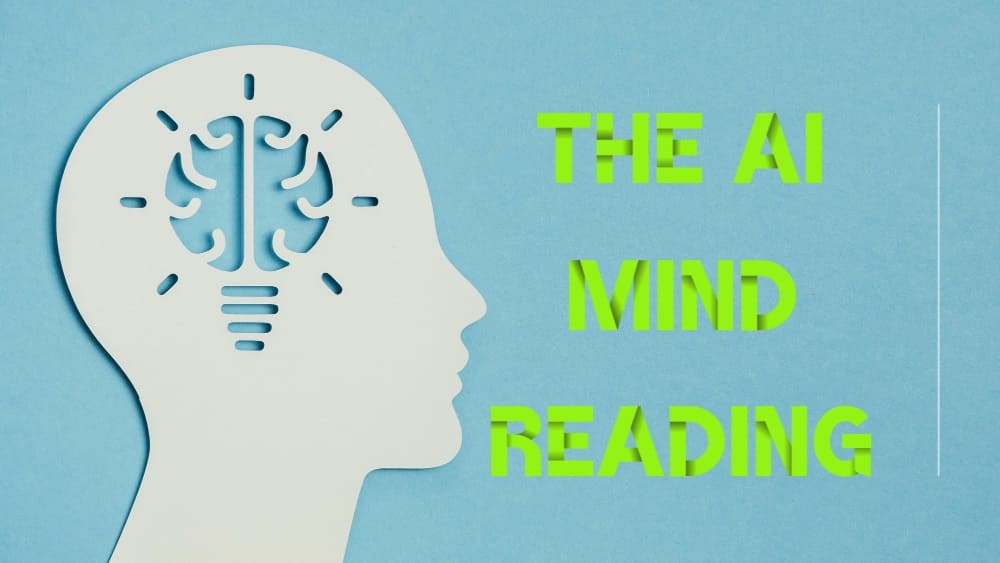
Overview:
AI mind-reading, once a science fiction concept, is becoming a reality by using advanced artificial intelligence to interpret human thoughts, emotions, and intentions from neural signals and physiological data. To understand what is AI Mind-Reading, we first have to learn about the technologies that capture brain activity through methods like Electroencephalography (EEG), Functional Magnetic Resonance Imaging (fMRI), and Near-Infrared Spectroscopy (NIRS). These technologies process the data with powerful AI algorithms to recognize patterns and predict cognitive states. These insights have transformative applications in neurorehabilitation, communication for individuals with disabilities, personalized learning, and immersive gaming.
However, the advancement of AI mind-reading is not without its challenges. Issues related to privacy, ethical use, accuracy, and regulatory compliance must be meticulously addressed to ensure the responsible development and deployment of these technologies. Protecting the confidentiality of neural data, obtaining informed consent, and adhering to stringent data protection regulations are paramount. Additionally, ethical guidelines must be established to prevent misuse and ensure the technology benefits society. This article delves into the workings of AI mind-reading, explores its key applications and benefits, and examines the potential hurdles and regulatory frameworks shaping its future.
Contents:
- What is AI Mind-Reading
- Key technologies enabling AI Mind-Reading capabilities
- How AI Mind-Reading mechanism works
- Prominent application areas of AI Mind-Reading
- Potential challenges with AI Mind-Reading that need to be addressed
- Key regulatory and compliance considerations in AI Mind-Reading
- Summing Up
So, what is AI Mind-Reading:
The AI mind-reading generally refers to the use of artificial intelligence technologies to interpret and predict human thoughts, intentions, or emotions. While the term “mind-reading” might suggest a futuristic or science-fiction scenario, current AI technologies are more about interpreting and predicting based on observable data rather than truly reading minds. Ethical considerations and privacy concerns are also significant aspects of this field, as the ability to interpret thoughts and emotions can lead to misuse if not properly regulated.
Key technologies enabling AI Mind-Reading capabilities:
1. Brain-Computer Interfaces (BCIs):
1.A Electroencephalography (EEG):
Uses electrodes placed on the scalp to detect electrical activity in the brain.
Commonly used in non-invasive BCIs due to their relatively low cost and ease of use.
Applications include neurofeedback therapy, controlling prosthetic limbs, and even gaming.
1.B Functional Magnetic Resonance Imaging (fMRI):
Uses electrodes placed on the scalp to detect electrical activity in the brain.
Commonly used in non-invasive BCIs due to their relatively low cost and ease of use.
Applications include neurofeedback therapy, controlling prosthetic limbs, and even gaming.
1.C Magnetoencephalography (MEG):
Measures the magnetic fields produced by neural activity using highly sensitive magnetometers.
Offers high temporal resolution, capturing rapid changes in brain activity.
Used in research and clinical diagnosis of neurological disorders.
1.D Near-Infrared Spectroscopy (NIRS):
Uses near-infrared light to measure changes in blood oxygenation and volume in the brain.
Non-invasive and portable, making it suitable for a variety of settings, including cognitive and developmental research.
2. Neural Decoding:
2.A Machine Learning Algorithms:
Algorithms such as Support Vector Machines (SVM), Random Forests, and k-Nearest Neighbors (k-NN) are trained to recognize patterns in brain activity data.
Used to classify different mental states, such as focus vs. relaxation, or to predict specific intentions.
2.B Deep Learning:
Deep neural networks, including Convolutional Neural Networks (CNNs) and Recurrent Neural Networks (RNNs), are employed to model complex relationships in brain data.
CNNs are effective for processing EEG data, while RNNs are suitable for time-series data like neural activity over time.
Used in advanced applications like interpreting imagined speech or visualizing thoughts.
2.C Sentiment Analysis:
Analyzes text to determine the emotional tone, such as positive, negative, or neutral sentiment.
Utilizes techniques like lexical analysis, parsing, and machine learning to understand context and subtleties.
Applied in social media monitoring, customer feedback analysis, and mental health assessments.
3. Emotion Recognition:
3.A Computer Vision:
Analyzes facial expressions to infer emotions using algorithms that detect key facial landmarks and movements.
Techniques include feature extraction, facial action coding systems (FACS), and deep learning for enhanced accuracy.
Applications range from market research to therapeutic interventions.
3.B Physiological Monitoring:
Uses data from wearable devices to monitor physiological indicators like heart rate variability, skin conductance, and respiratory rate.
Provides insights into stress levels, arousal, and emotional responses.
Applied in fitness tracking, mental health monitoring, and stress management.
4. Wearable Technology:
4.A Smartwatches and Fitness Trackers:
Collect physiological data such as heart rate, steps taken, sleep patterns, and activity levels.
Equipped with sensors like accelerometers, gyroscopes, and optical heart rate monitors.
Integrated with apps that analyze data to provide health insights and recommendations.
4.B EEG Headsets:
Portable devices that allow for real-time monitoring of brain activity outside of clinical or lab settings.
Used in applications ranging from meditation and concentration training to gaming and neurofeedback.
Examples include Muse, Emotiv, and NeuroSky.
5. Data Analytics:
5.A Big Data:
Involves the collection and analysis of large datasets to identify patterns, correlations, and trends.
Utilizes technologies like Hadoop, Spark, and data warehouses to handle vast amounts of data efficiently.
Applied in behavior prediction, and user experience optimization.
5.B Predictive Analytics:
Uses statistical techniques and machine learning to predict future behavior or outcomes based on historical data.
Involves regression analysis, classification algorithms, and time-series forecasting.
Now, let’s understand how AI Mind-Reading mechanism works:
AI Mind-Reading working mechanism involves interpreting and understanding human thoughts, intentions, or emotions using artificial intelligence technologies. Here’s a high-level overview of how AI mind-reading works:
1. Data Acquisition:
Neural Signals: AI mind-reading typically begins with capturing neural signals from the brain. This can be done using various methods such as EEG (Electroencephalography), fMRI (Functional Magnetic Resonance Imaging), MEG (Magnetoencephalography), or NIRS (Near-Infrared Spectroscopy).
Physiological Data: In addition to neural signals, physiological data such as heart rate variability, skin conductance, or facial expressions may also be collected to provide context about the individual’s emotional and cognitive state.
2. Preprocessing and Feature Extraction:
Signal Processing: Raw neural data undergoes preprocessing to remove noise and artifacts, enhancing the quality of signals for analysis.
Feature Extraction: Relevant features, such as frequency bands in EEG or activation patterns in fMRI, are extracted from the preprocessed data. These features serve as inputs for AI algorithms.
3. AI Algorithms and Models:
Various machine learning techniques are applied to interpret neural and physiological data. These may include:
Supervised Learning: Training models with labeled data to classify brain states or emotional responses.
Unsupervised Learning: Identifying patterns and structures in neural data without predefined labels.
Deep Learning: Using neural networks to learn complex patterns and relationships in data, particularly effective in tasks like image analysis (fMRI) or time-series data (EEG).
4. Interpretation and Prediction:
Pattern Recognition: Trained AI models analyze extracted features to recognize patterns associated with specific thoughts, emotions, or intentions.
Prediction: Based on learned patterns, AI systems can predict a person’s intended action, emotional state, or response to stimuli.
5. Feedback and Interaction:
Closed-loop Systems: In some applications, AI mind-reading systems may provide real-time feedback or interact with the user based on their neural and physiological signals.
Applications: This technology finds applications in healthcare (neurorehabilitation, mental health), communication (assistive devices), education (adaptive learning), entertainment (interactive experiences), and more.
Prominent application areas of AI Mind-Reading:
1. General Health – Neurorehabilitation:
AI mind-reading powered BCIs help stroke and spinal cord injury patients regain motor function by translating brain signals into movements, aiding in physical therapy.
Example: Mind-controlled prosthetics and exoskeletons that help patients perform daily tasks and undergo rehabilitation exercises.
2. Mental Health – Emotion Monitoring and Regulation:
AI mind-reading systems analyze physiological and neural data to detect emotional states, providing feedback for mental health monitoring and interventions.
Example: Wearable devices that monitor stress levels and provide real-time feedback or interventions, such as guided breathing exercises.
3. Communication – Assistive Communication Devices:
AI mind-reading technologies, such as BCIs and neural decoding, enable individuals with speech or motor impairments to communicate by translating their thoughts into text or speech.
Example: Brain-controlled typing systems for individuals with ALS or severe paralysis, allowing them to communicate more effectively.
4. Education – Personalized Learning:
AI mind-reading driven emotion and attention tracking systems personalize learning experiences based on students’ cognitive and emotional states.
Example: Adaptive learning platforms that adjust difficulty levels and content delivery based on real-time analysis of students’ engagement and stress levels.
5. Entertainment – Immersive Gaming Experiences:
AI mind-reading BCIs and emotion recognition technologies create more immersive and interactive gaming experiences by responding to players’ thoughts and emotions.
Example: Games that adjust difficulty, storyline, or gameplay elements based on the player’s emotional and cognitive state, enhancing engagement and personalization.
Potential challenges with AI Mind-Reading that need to be addressed:
1. Privacy and Security:
Data Privacy:
The collection and analysis of brain activity data raise significant privacy concerns. Ensuring that sensitive neural data is protected and used ethically is paramount.
Data Security:
Safeguarding neural data from unauthorized access and cyber-attacks is crucial to prevent misuse and protect individuals’ mental privacy.
2. Ethical Concerns:
Informed Consent:
Obtaining clear and informed consent from individuals whose brain data is being collected and analyzed is essential. Users must fully understand how their data will be used.
Potential for Misuse:
The technology could be misused for surveillance, manipulation, or coercion, raising ethical questions about the limits and controls on AI mind-reading applications.
3. Accuracy and Reliability:
Interpretation of Neural Data:
Decoding complex thoughts, emotions, and intentions accurately remains a significant challenge. Misinterpretations can lead to incorrect outcomes or decisions.
Variability Among Individuals:
Brain activity patterns can vary widely between individuals, making it difficult to develop universally accurate AI mind-reading systems.
4. Technical Challenges:
Signal Quality and Noise:
Neural signals can be noisy and subject to interference from various sources, complicating the process of accurate data interpretation.
Integration with Existing Systems:
Seamlessly integrating AI mind-reading technologies with existing devices and systems requires overcoming technical and compatibility hurdles.
5. Cost and Accessibility:
High Development and Implementation Costs:
The advanced technology and infrastructure required for AI mind-reading can be costly, potentially limiting access to well-funded institutions or individuals.
Equity and Inclusion:
Ensuring that AI mind-reading technologies are accessible to diverse populations and do not exacerbate existing inequalities is a significant challenge.
6. Legal Issues
Lack of Standards:
The rapid development of AI mind-reading technologies has outpaced the establishment of regulatory standards and guidelines, leading to potential legal uncertainties.
Intellectual Property:
Determining ownership and rights over neural data and AI models that interpret this data can be legally complex.
7. Social and Psychological Impact:
User Trust and Acceptance:
Gaining user trust and acceptance of AI mind-reading technologies is crucial. Concerns about privacy, accuracy, and ethical use may hinder widespread adoption.
Impact on Human Interaction:
The pervasive use of AI mind-reading could alter the nature of human interactions and raise questions about the balance between human autonomy and technological control.
Key regulatory and compliance considerations in AI Mind-Reading:
Regulations and compliance for AI mind-reading technologies are still evolving, as the field is relatively new and rapidly advancing. However, several key areas are being addressed by existing and emerging regulations:
1. Data Privacy and Protection:
General Data Protection Regulation (GDPR):
Applicable in the European Union, GDPR provides stringent rules on data protection and privacy. It requires explicit consent from users for data collection, the right to access and erase their data, and mandates data protection measures.
California Consumer Privacy Act (CCPA):
In the United States, the CCPA grants California residents rights over their personal data, including the right to know what data is being collected, the right to delete their data, and the right to opt out of the sale of their data.
2. Medical Device Regulation:
FDA (Food and Drug Administration):
In the United States, the FDA regulates medical devices, including those that incorporate AI for healthcare applications. AI mind-reading devices used for medical purposes must meet stringent safety and efficacy standards.
European Medical Device Regulation (MDR):
In the EU, the MDR ensures that medical devices, including AI-based systems, meet high standards for safety and performance before they can be marketed.
3. Ethical Guidelines:
IEEE Global Initiative on Ethics of Autonomous and Intelligent Systems:
The IEEE has developed ethical guidelines for the design and implementation of AI systems, emphasizing transparency, accountability, and the protection of human rights.
AI Ethics Guidelines by National and International Bodies:
Various countries and organizations, including UNESCO and the European Commission, have issued guidelines and frameworks for ethical AI, addressing issues such as fairness, accountability, and transparency.
4. Neurotechnology-Specific Regulations:
Neuroethics:
Emerging neuroethics frameworks focus on the ethical implications of neurotechnology, including AI mind-reading. These frameworks address issues such as cognitive liberty, mental privacy, and the potential for misuse.
National Institutes of Health (NIH):
In the US, the NIH has guidelines for research involving neural data and neurotechnologies, ensuring ethical considerations are incorporated into scientific studies.
5. Industry Standards and Best Practices:
ISO/IEC JTC 1/SC 42:
ISO/IEC JTC 1/SC 42 (International Organization for Standardization/International Electrotechnical Commission Joint Technical Committee on Artificial Intelligence) develops standards for AI, including those related to data quality, trustworthiness, and governance, which can be applied to AI mind-reading technologies.
Best Practices for Data Handling:
Industry best practices for data handling, including anonymization, encryption, and secure storage, are critical for protecting the sensitive neural data involved in AI mind-reading.
6. Informed Consent and User Rights:
Informed Consent Regulations:
Regulations in medical and research contexts require obtaining informed consent from individuals before collecting and using their neural data. This includes explaining the purpose, risks, and benefits of data collection.
User Rights Legislation:
Laws ensuring that users have rights over their neural data, including the right to access, correct, and delete their data, and to understand how it is being used.
7. Intellectual Property and Ownership:
IP Laws:
Intellectual property laws protect the innovations in AI mind-reading technologies, but also raise questions about the ownership of neural data and AI models derived from this data.
Summing Up:
AI mind-reading stands at the frontier of technological innovation, promising to revolutionize how we interact with machines and understand the human mind. Its applications span numerous sectors, from healthcare and communication to education and entertainment, offering unprecedented opportunities for personalized and responsive experiences. By interpreting neural signals and physiological data, AI mind-reading technologies can enhance quality of life, improve accessibility, and provide new tools for mental health and cognitive research.
However, the development and deployment of AI mind-reading must be approached with caution. Addressing privacy concerns, ensuring data security, and establishing ethical guidelines are crucial to prevent misuse and protect individuals’ mental privacy. Additionally, rigorous regulatory frameworks are necessary to ensure the safety and efficacy of these technologies. As we navigate the challenges and possibilities of AI mind-reading, a collaborative effort from technologists, ethicists, policymakers, and society at large will be essential to harness its potential responsibly and beneficially.
Courtesy Image: FreePik