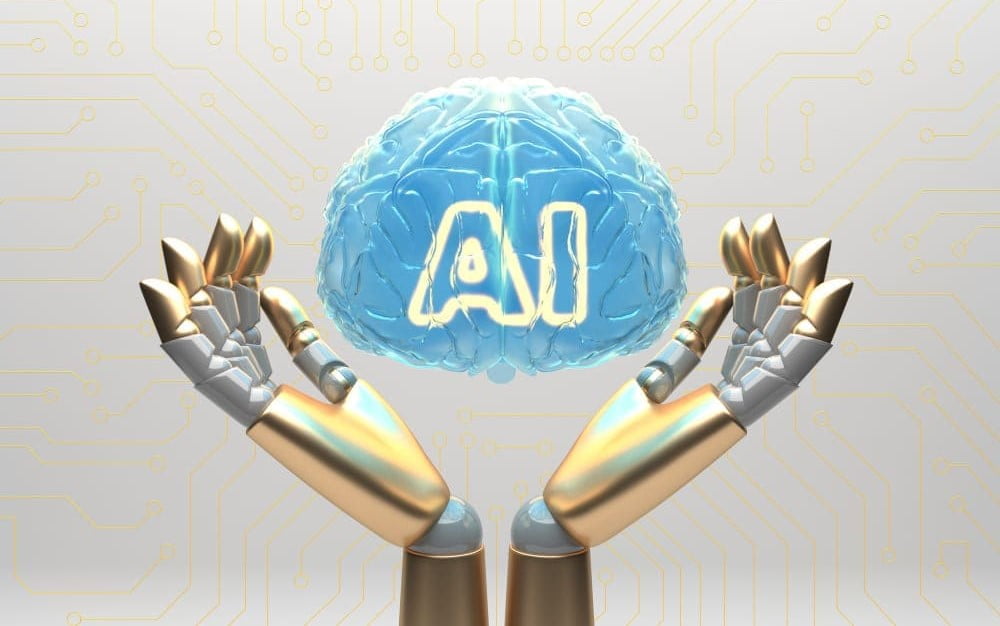
Overview:
Artificial Intelligence (AI) is transforming our world at an unprecedented pace, offering remarkable advancements across numerous domains. From healthcare to finance, AI’s potential to improve efficiency, accuracy, and overall quality of life is undeniable. However, with great power comes great responsibility. The development and deployment of AI technologies bring forth significant ethical considerations, making Responsible AI a critical focal point for researchers, developers, policymakers, and society at large. What is Responsible AI? It encompasses the principles and practices that ensure AI systems are developed and used in ways that are ethical, transparent, and beneficial to all.
Responsible AI further, involves addressing key aspects such as bias reduction, accountability, privacy, and inclusivity to create AI systems that are not only effective but also equitable and trustworthy. As we navigate the complexities of integrating AI into various facets of our lives, it is essential to understand both the benefits and the challenges associated with Responsible AI, as well as envisioning its future trajectory.
Contents:
- What is Responsible AI
- Key Principles of Responsible AI
- Key technologies enabling Responsible AI
- Key Application Areas of Responsible AI
- Key Benefits of Responsible AI
- Key Challenges with the Implementation of Responsible AI
- The Futuristic Outlook of Responsible AI
- Summing Up
So, what is Responsible AI (RAI):
Responsible AI refers to the practice of designing, developing, and deploying artificial intelligence (AI) systems in a manner that is ethical, transparent, and accountable. This involves ensuring that AI technologies are fair, reliable, safe, and respectful of user privacy and societal norms.
Key Principles of Responsible AI:
1. Fairness:
Ensuring that AI systems do not perpetuate biases or discrimination. This involves using unbiased data, designing algorithms that treat all users equally, and regularly auditing AI outcomes to identify and correct any unfair practices.
Example: Imagine an AI used in hiring that reviews resumes. If it’s responsible, it won’t favor one gender or race over another. Instead, it will focus only on qualifications and experience.
2. Transparency:
Making AI systems and their decision-making processes understandable and explainable to users and stakeholders. This includes providing clear documentation, explaining how decisions are made, and allowing for scrutiny by third parties.
Example: If you use an app that suggests songs or movies, transparency means the app explains why it recommended those choices based on your past preferences, not just giving random suggestions.
3. Accountability:
Establishing clear lines of responsibility for AI systems and their outcomes. This includes having mechanisms in place for addressing harm or negative consequences caused by AI, and ensuring that there are humans in the loop who can intervene when necessary.
Example: If an AI in a self-driving car makes a mistake and causes an accident, there should be clear guidelines on who is responsible for investigating and fixing the problem, whether it’s the manufacturer or the software developer.
4. Privacy and Security:
Protecting user data and ensuring that AI systems comply with relevant data protection regulations. This involves implementing robust security measures and designing systems that minimize data collection and use data responsibly.
Example: When you use a health app that tracks your exercise and diet, responsible AI ensures your data is kept private and secure, and not shared without your permission.
5. Reliability and Safety:
Ensuring that AI systems perform consistently and accurately, and do not cause harm to users or society. This includes rigorous testing, validation, and monitoring of AI systems in real-world conditions.
Example: In medical diagnosis, an AI that helps doctors identify diseases from X-rays must be highly accurate and thoroughly tested to ensure it doesn’t give false results that could harm patients.
6. Inclusiveness:
Ensuring that AI benefits all segments of society and does not exclude or disadvantage any group. This involves engaging diverse stakeholders in the design and deployment of AI systems.
Example: Voice recognition systems should understand different accents and dialects, so they work well for everyone, not just people with standard accents.
7. Environmental and Social Well-being:
Considering the broader impacts of AI on the environment & society and striving to develop sustainable and socially beneficial AI applications.
Example: An AI that helps reduce energy consumption in smart homes contributes to environmental well-being by lowering electricity use and reducing carbon footprints.
Key technologies enabling Responsible AI:
1. Fairness and Bias Detection Tools:
Example-1: IBM’s AI Fairness 360 is a comprehensive toolkit that helps developers check their models for biases and provides metrics and algorithms to mitigate them.
Example-2: Microsoft’s Fairlearn is another toolkit that assesses and improves the fairness of AI systems.
2. Explainability and Interpretability Technologies:
Example-1: LIME (Local Interpretable Model-agnostic Explanations) helps in understanding the decisions of complex models by approximating them locally with simpler, interpretable models.
Example-2: SHAP (SHapley Additive exPlanations) values explain individual predictions by attributing the contribution of each feature to the prediction.
3. Privacy-Preserving Techniques:
Example-1: Differential Privacy adds random noise to data in a way that statistical analyses can still be performed while ensuring that individual data points cannot be identified.
Example-2: Federated Learning allows AI models to be trained across multiple devices or servers holding local data samples, without exchanging them. This keeps the data decentralized and more secure.
4. Security Technologies:
Example-1: Homomorphic Encryption allows computations to be performed on encrypted data without needing to decrypt it first, enhancing data security.
Example-2: Secure Multi-Party Computation (SMPC) allows multiple parties to jointly compute a function over their inputs while keeping those inputs private.
5. Auditing and Monitoring Tools:
Example-1: Model Monitoring tools like Fiddler or WhyLabs continuously track the performance of AI models to ensure they behave as expected over time and under different conditions.
Example-2: Ethical AI checklists and frameworks guide developers through ethical considerations during the AI development lifecycle.
6. Robustness and Reliability Technologies:
Example-1: Adversarial Training involves exposing AI models to adversarial examples during training to make them more robust against malicious attacks.
Example-2: Red Teaming exercises involve ethical hacking techniques to identify vulnerabilities in AI systems.
7. Inclusiveness and Accessibility Tools:
Example-1: Inclusive design tools and guidelines help create AI systems that are accessible to people with disabilities, such as voice recognition systems that understand different accents and speech patterns.
Example-2: Text-to-speech and speech-to-text technologies that cater to diverse user needs, ensuring broad usability.
8. Environmental and Ethical Impact Assessments:
Example-1: Lifecycle analysis tools assess the environmental impact of AI systems from development through deployment and decommissioning.
Example-2: Ethical AI frameworks and guidelines, such as those from the IEEE and European Commission, help ensure that AI development considers broader societal impacts.
Key Application Areas of Responsible AI:
1. Healthcare:
Example-1: AI for medical diagnostics can identify diseases from medical images. Responsible AI ensures these systems are accurate, explainable, and unbiased across different demographics to avoid disparities in healthcare outcomes.
Example-2: Predictive analytics for patient care plans, ensuring patient data privacy and security through technologies like differential privacy.
2. Finance:
Example-1: AI in credit scoring can evaluate loan applications. Responsible AI ensures that these systems do not discriminate based on race, gender, or socioeconomic status and are transparent about decision criteria.
Example-2: Fraud detection systems that protect users’ financial information while maintaining high accuracy and minimizing false positives.
3. Hiring and Human Resources:
Example-1: AI-driven recruitment tools that screen resumes and conduct initial interviews. Responsible AI ensures these tools do not perpetuate biases and are transparent about the selection criteria.
Example-2: Employee monitoring systems that respect privacy and provide clear insights into how data is used.
4. Education:
Example-1: Personalized learning platforms that adapt to individual student needs. Responsible AI ensures these platforms are inclusive and accessible to students with diverse learning abilities and backgrounds.
Example-2: AI-driven grading systems that provide fair and unbiased assessments of student work.
5. Law Enforcement and Public Safety:
Example-1: Predictive policing tools that help allocate resources to high-crime areas. Responsible AI ensures these tools do not reinforce existing biases and are transparent and accountable.
Example-2: AI for surveillance and facial recognition that respects privacy rights and is used with proper ethical guidelines.
6. Customer Service:
Example-1: AI chatbots and virtual assistants that provide customer support. Responsible AI ensures these systems are transparent when users are interacting with AI, and that data is securely handled.
Example-2: Sentiment analysis tools that help understand customer emotions while respecting user privacy.
7. Marketing and Advertising:
Example-1: AI for targeted advertising that tailors ads to individual preferences. Responsible AI ensures user data is used ethically and transparently, with options for users to control their data.
Example-2: Content recommendation systems that are fair and do not create echo chambers or promote harmful content.
8. Transportation:
Example-1: Autonomous vehicles that navigate and drive. Responsible AI ensures these systems are safe, reliable, and operate transparently regarding decision-making processes in critical situations.
Example-2: Traffic management systems that optimize flow and reduce congestion while ensuring data privacy and security.
9. Environment and Sustainability:
Example-1: AI for climate modeling and predicting environmental changes. Responsible AI ensures models are accurate, reliable, and used to make informed policy decisions.
Example-2: Smart grids and energy management systems that optimize energy use and reduce waste, with considerations for environmental impacts.
10. Governance and Public Policy:
Example-1: AI in public administration for resource allocation and policy implementation. Responsible AI ensures transparency, accountability, and fairness in decision-making processes.
Example-2: Civic engagement platforms that use AI to analyze public opinion and engage citizens, ensuring inclusivity and data privacy.
Key Benefits of Responsible AI:
1. Enhanced Trust and Acceptance:
- By being transparent and fair, AI systems can gain the trust of users and stakeholders.
Example: When an AI-based hiring tool explains its decisions clearly, job applicants are more likely to trust and accept the outcomes.
2. Improved Accountability and Compliance:
- Clearly defined accountability mechanisms ensure that AI systems comply with laws and ethical standards.
Example: Healthcare AI that adheres to strict privacy regulations and ethical guidelines enhances patient trust and legal compliance.
3. Data Privacy and Security:
- Protecting user data reduces the risk of breaches and misuse.
Example: AI-driven marketing platforms that prioritize data privacy help maintain customer trust and avoid legal penalties.
4. Increased Reliability and Safety:
- Rigorous testing and monitoring ensure AI systems perform reliably and safely.
Example: Autonomous vehicles designed with Responsible AI principles are more likely to navigate safely and handle unexpected situations effectively.
5. Greater Inclusivity and Accessibility:
- Designing AI systems to be inclusive ensures they benefit a broader range of users.
Example: Educational AI tools that cater to diverse learning needs and languages can help all students succeed.
6. Social and Environmental Benefits:
- AI systems designed with societal and environmental considerations can promote sustainability and social good.
Example: AI in smart grids that optimizes energy usage helps reduce carbon footprints and supports environmental sustainability.
7. Ethical Use of Technology:
- Ensuring ethical practices in AI development and deployment fosters a culture of responsibility.
Example: AI used in law enforcement that adheres to ethical guidelines helps protect civil liberties and promotes justice.
8. Enhanced Decision-Making:
- AI systems that are transparent and explainable aid better decision-making by providing clear insights and rationale.
Example: Medical AI that explains its diagnostic process helps doctors make informed treatment decisions.
9. Economic and Operational Efficiency:
- Responsible AI can optimize operations while maintaining ethical standards, leading to economic benefits.
Example: AI in supply chain management that ensures fair labor practices while optimizing logistics can reduce costs and improve brand reputation.
10. Regulatory and Market Advantage:
- Companies that adopt Responsible AI practices may gain a competitive edge and regulatory favor.
Example: Financial institutions using AI responsibly can enhance their reputation and attract customers who value ethical practices.
Key Challenges with the Implementation of Responsible AI:
1. Interpreting Complex AI Systems:
- Many advanced AI models, such as deep neural networks, are highly complex and operate as black boxes, making it difficult for stakeholders to understand how decisions are made.
Example: In sectors like healthcare or finance, where decisions impact individuals’ lives or financial outcomes, the lack of transparency can hinder trust and acceptance of AI systems.
2. Dynamic and Evolving Ethical Standards:
- Ethical guidelines for AI are continually evolving, making it challenging for developers to ensure their systems remain compliant with the latest standards.
Example: Issues such as AI-powered surveillance or facial recognition raise ethical concerns that require ongoing reassessment and adaptation of AI practices.
3. Ensuring Fairness Across Diverse Populations:
- Achieving fairness in AI systems across diverse demographics and cultural contexts can be challenging due to variations in data availability and societal norms.
Example: AI used in criminal justice systems must account for biases that may disproportionately affect certain demographic groups, requiring careful consideration of data selection and algorithmic design.
4. Data Quality Maintenance:
- Ensuring high-quality data for training AI models is crucial but often difficult to achieve, especially when historical data reflects societal biases.
Example: Bias in datasets used for training AI in hiring practices can perpetuate discrimination if not properly identified and corrected through robust data preprocessing techniques.
5. Human-AI Collaboration and Trust:
- Establishing effective collaboration between AI systems and human decision-makers requires overcoming challenges related to trust, communication, and shared decision-making processes.
Example: Autonomous systems in healthcare, where AI assists doctors in diagnosis and treatment decisions, must navigate challenges in integrating AI insights with medical expertise while maintaining patient trust.
6. Adapting to Regulatory and Legal Frameworks:
- Compliance with diverse regulatory frameworks globally poses a significant challenge for organizations developing and deploying AI technologies.
Example: Cross-border data transfer regulations, such as GDPR in Europe and CCPA in California, require careful navigation to ensure AI systems meet legal requirements while respecting user privacy.
7. AI System Bias Detection and Mitigation:
- Developing effective tools and methodologies for detecting and mitigating biases in AI systems remains a technical and operational challenge.
Example: Bias detection tools may not always accurately identify subtle biases, requiring continuous improvement and validation against diverse datasets and real-world scenarios.
8. Scalability and Integration with Existing Systems:
- Integrating Responsible AI practices into existing organizational systems and workflows while maintaining scalability can be complex and resource-intensive.
Example: Upgrading legacy systems with AI capabilities requires balancing technical requirements with operational constraints to ensure smooth integration and performance.
9. Education and Skills Gap:
- Addressing the gap in AI literacy and ethical training among developers, stakeholders, and users is essential for fostering responsible AI practices.
Example: Ensuring that developers and decision-makers understand the ethical implications of AI decisions and have the skills to implement ethical guidelines effectively.
10. Societal Impact and Public Perception:
- Managing public perceptions and concerns about AI’s societal impact, such as job displacement or loss of human decision-making autonomy, requires proactive communication and engagement.
Example: Public debates around AI’s role in employment and privacy can influence policy decisions and public acceptance, necessitating transparent communication and engagement with stakeholders.
Before closing, Let’s explore the Futuristic Outlook of Responsible AI:
1. Advanced Quantum AI for Ethical Decision-Making:
- Quantum computing will enhance AI capabilities, allowing for more sophisticated and faster ethical decision-making processes.
Example: Quantum AI systems in healthcare could analyze vast amounts of genetic data in real-time to provide ethical, personalized treatment plans for patients, considering both medical efficacy and ethical implications.
2. Self-Regulating AI Systems:
- AI systems will become self-regulating, equipped with built-in mechanisms to monitor and adjust their operations to ensure ethical compliance autonomously.
Example: AI-powered financial systems will continuously self-audit and correct biases in lending practices without human intervention, ensuring fairness and transparency.
3. Neural Interfaces for Seamless Human-AI Interaction:
- Brain-computer interfaces (BCIs) will enable direct communication between humans and AI, fostering seamless and intuitive collaboration.
Example: BCIs could allow users to control and interact with AI assistants using their thoughts, enhancing accessibility for individuals with disabilities and creating new possibilities for human-AI partnerships.
4. AI-Driven Ethical Governance Platforms:
- Decentralized AI governance platforms will use blockchain and AI to ensure transparent, democratic decision-making processes for AI regulations and policies.
Example: Global AI policies will be developed and managed through a decentralized platform where stakeholders can vote on ethical guidelines and monitor AI compliance in real-time.
5. AI-Augmented Reality (AR) for Ethical Training:
- AI-enhanced AR environments will provide immersive ethical training for professionals across various fields.
Example: Medical professionals could use AI-powered AR simulations to practice complex surgeries ethically, receiving real-time feedback on their decisions and actions.
6. Autonomous AI Ethics Auditors:
- Independent AI entities will serve as ethics auditors, continuously evaluating and ensuring that other AI systems adhere to ethical standards.
Example: These autonomous auditors could monitor AI applications in sensitive areas like criminal justice, providing unbiased assessments and recommendations for improvement.
7. Global AI Ethics Councils:
- AI-driven global ethics councils will provide real-time guidance and resolutions for ethical dilemmas faced by AI systems worldwide.
Example: An AI ethics council could quickly address and resolve issues related to autonomous weapons, ensuring they operate within internationally agreed ethical frameworks.
8. AI-Enhanced Environmental Stewardship:
- AI will play a pivotal role in environmental conservation, optimizing resource use and monitoring ecological impacts with unprecedented precision.
Example: AI-powered drones and satellites will monitor deforestation and wildlife populations in real-time, making data-driven recommendations to governments and NGOs for sustainable practices.
9. Synthetic Data for Ethical AI Training:
- AI systems will increasingly use high-quality synthetic data to train models, ensuring privacy and reducing biases associated with real-world data.
Example: Autonomous vehicles will be trained using diverse and realistic synthetic data sets, ensuring they perform safely and fairly across different environments and conditions.
10. AI-Driven Social Justice Advocates:
- AI systems will actively advocate for social justice, identifying and addressing systemic inequalities in real-time.
Example: AI could analyze social media and public data to detect and highlight discriminatory practices in real-time, providing actionable insights to policymakers and activists.
11. Personalized Ethical AI Assistants:
- Personalized AI assistants will help individuals navigate ethical dilemmas in their daily lives, offering tailored advice based on a deep understanding of personal values and societal norms.
Example: An AI assistant could help users make ethical decisions about their digital footprint, providing suggestions on data sharing and privacy settings aligned with their values.
Summing Up:
In summary, the journey towards Responsible AI is marked by both significant benefits and formidable challenges, each crucial for the ethical integration of AI into society. As AI technologies continue to evolve, their potential to enhance trust, fairness, and efficiency across various sectors becomes increasingly evident. However, addressing issues such as bias, transparency, accountability, and inclusivity remains imperative to ensure these systems are both ethical and effective.
Looking forward, the future of Responsible AI is promising and dynamic, with advancements in quantum computing, neural interfaces, and autonomous ethical auditors poised to revolutionize the field. These innovations will facilitate more sophisticated ethical decision-making, seamless human-AI collaboration, and robust self-regulation mechanisms, all while promoting sustainability and social justice.
As we embrace these futuristic developments, it is essential to foster interdisciplinary collaboration, global cooperation, and continuous adaptation to ethical standards. By doing so, we can harness the full potential of AI technologies in a manner that is not only beneficial and efficient but also fundamentally ethical and responsible. Through a concerted effort from all stakeholders, we can ensure that AI serves as a force for good, driving positive change and enhancing the well-being of individuals and society as a whole.
One thought on “What is Responsible AI (RAI)? Uncover its Ethical Considerations and More”