
Overview:
AI Cloud, or Artificial Intelligence Cloud, represents an innovative integration of technologies that empowers organizations to harness AI capabilities within cloud infrastructure similar to edge computing. This integration seamlessly merges cloud computing with artificial intelligence (AI), enabling organizations to integrate AI tools, algorithms, and cloud services into their everyday operations. It provides advanced capabilities for managing data analytics, predictions, natural language processing, recommendations, and more. In this article, we’ll dive deeper into its various aspects, so let’s move in together and explore further.
Contents:
- What is AI Cloud
- What AI Cloud Ecosystem Looks Like
- Key Components of AI Cloud Ecosystem
- The AI Cloud Market Landscape
- Key Developments in AI Cloud
- Key Driving Forces of AI Cloud Growth
- Key Application Areas of AI Cloud
- How AI Cloud Solves Business Problems
- Potential Challenges that Come with AI Cloud
What is AI in the Cloud (AI Cloud):
The integration of cloud computing and AI, referred to as Artificial Intelligence Cloud or AI Cloud, represents a convergence of technologies. By merging AI with cloud computing, organizations can seamlessly integrate AI tools, algorithms, and cloud services into their daily operations. AI Cloud empowers enterprises to harness the full potential of AI, including machine learning, natural language processing, and computer vision, providing them with a competitive edge.
One of the primary advantages of AI Cloud is its capacity to rapidly and efficiently process extensive datasets. This capability is particularly beneficial in data-centric industries such as e-commerce, banking, and healthcare, enabling machine learning algorithms to uncover hidden patterns and insights within large data repositories. By making decisions based on data-driven insights, organizations can enhance their strategic decision-making processes.
Furthermore, AI Cloud facilitates the automation of tedious and repetitive tasks through the deployment of AI-powered algorithms. This automation streamlines operations, increases efficiency, and reduces dependence on manual labor, resulting in significant cost savings and productivity gains. The real-time capabilities of AI Cloud are instrumental in various applications, from enabling swift customer service through chatbots to automating production processes, illustrating its transformative impact across different sectors and enterprises.
By leveraging the scalability, flexibility, and accessibility of cloud computing, AI-powered organizations can thrive in a dynamic and responsive corporate ecosystem. This integration positions businesses advantageously within the data-driven, AI-enhanced business landscape, fostering innovation and growth.
What AI Cloud Ecosystem Looks Like:
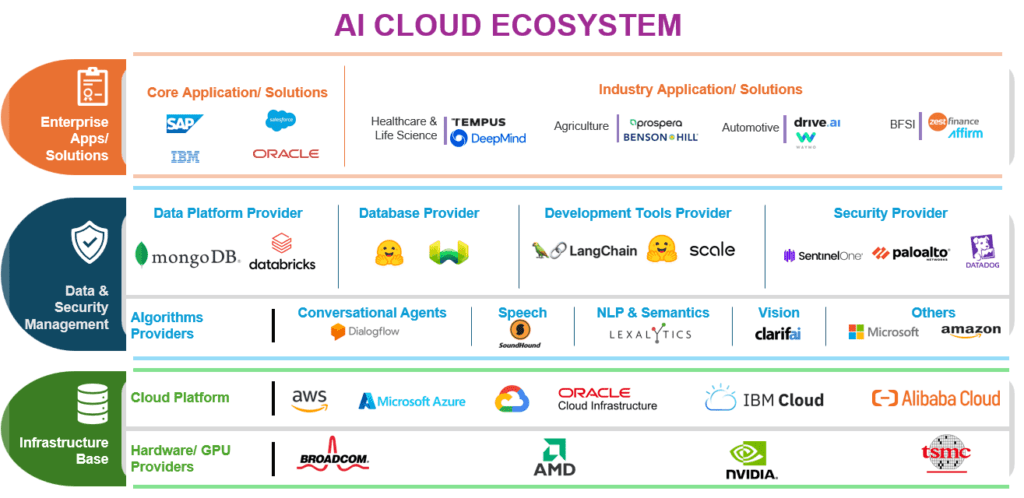
Key Components of AI Cloud Ecosystem:
Infrastructure Base:
The foundation of AI cloud infrastructure comprises two primary components: the cloud platform and the hardware. These components are fundamental to the AI Cloud ecosystem as they form the backbone upon which everything else relies.
Hardware, such as GPUs (Graphics Processing Units), plays a crucial role by providing the computational power needed to process vast amounts of data efficiently. When integrated with cloud computing environments, hardware components like GPUs establish the infrastructure necessary for running diverse applications and managing various types of data.
The cloud platform serves as the framework that facilitates the deployment, management, and scaling of AI applications and services. It provides the necessary resources, including storage, processing power, and networking capabilities, to support the execution of AI tasks.
Together, the cloud platform and hardware components form a robust foundation for AI cloud infrastructure, enabling organizations to leverage advanced AI technologies effectively. This infrastructure empowers businesses to harness the potential of AI for tasks ranging from data analysis and machine learning to natural language processing and computer vision.
Data and Security Management:
Another crucial aspect of the AI Cloud ecosystem is the management of data and security, particularly concerning critical data inputs and outputs. This sub-segment encompasses various elements, including database providers, data platform providers, development tools providers, security providers, and key algorithm providers for speech, vision, and conversation.
Data management involves handling the vast amounts of data generated and processed within the AI Cloud infrastructure. Database providers offer solutions for storing and organizing data efficiently, ensuring accessibility and reliability. Data platform providers contribute tools and frameworks for managing and analyzing data, enabling organizations to derive valuable insights from their datasets.
Security is paramount in safeguarding sensitive information and ensuring the integrity of AI processes. Security providers offer solutions for protecting data against unauthorized access, breaches, and cyber threats. They implement measures such as encryption, access controls, and threat detection to mitigate risks and maintain compliance with regulatory requirements.
Furthermore, development tools providers offer platforms and utilities for building and deploying AI applications, streamlining the development lifecycle and facilitating collaboration among teams. Key algorithm providers specialize in developing algorithms for speech recognition, computer vision, natural language processing, and other AI tasks, enhancing the capabilities of AI systems.
Together, data and security management form an integral part of the AI Cloud ecosystem, enabling organizations to harness the power of AI while ensuring the confidentiality, integrity, and availability of their data assets.
Applications and Solutions:
One of the final but crucial sub-components of the AI cloud ecosystem is the end-user applications and solutions. These encompass applications designed for various organizational functions, including sales and marketing, human resources, finance, operations, and more. Additionally, industry-specific applications cater to standardized procedures across entire sectors.
These applications are tailored to address specific needs and challenges within organizations, enhancing efficiency, productivity, and decision-making processes. They leverage AI technologies to automate tasks, analyze data, and provide insights that drive informed decision-making.
Furthermore, there are specialized players within the industry who develop these applications to target specific user groups. These solutions are often customized to meet the unique requirements of their intended users, providing tailored functionalities and features.
Moreover, the end-user applications and solutions play a pivotal role in the AI cloud ecosystem by empowering organizations with tools to optimize their operations and achieve their strategic objectives efficiently.
The AI Cloud Market Landscape:
According to the 2023 IDC report commissioned by Salesforce, the AI Cloud market is projected to experience substantial growth, increasing from USD 64 billion in 2023 to USD 222.4 billion by 2027, representing a remarkable compound annual growth rate (CAGR) of 36.5% over the period. This growth trajectory reflects the increasing adoption of AI technologies integrated within cloud infrastructure by enterprises worldwide. To expedite their digital transformation endeavors, organizations are embracing both managed AI services and self-hosted AI solutions within cloud environments.
A comprehensive study conducted by Wiz Research sheds light on the adoption trends of AI services and tools among cloud customers utilizing major cloud service providers (CSPs). The findings reveal a diverse landscape of adoption levels: while 32% of organizations are in the early stages, experimenting with fewer than 10 instances, 28% are actively leveraging managed AI services, and 10% are identified as power users, deploying 50 or more instances.
Furthermore, organizations are not solely reliant on managed AI services provided by CSPs; many are also harnessing the potential of self-hosted AI models within their internal environments. Notably, popular AI models such as BERT and DistilBERT are gaining traction, with 49% and 24% adoption rates respectively, underscoring the diverse strategies employed by organizations to train AI models and derive actionable insights
Key Developments in AI Cloud:
Growing Alliances in the AI Community:
Specialized AI service vendors are uniting to create advanced and comprehensive services, aiming to gain a competitive advantage in the AI cloud market. Oracle, for instance, teamed up with LangChain, an open-source AI framework provider, to facilitate seamless Oracle database queries using Large Language Models (LLMs) and to craft AI-driven business applications. Similarly, Google Cloud collaborated with Kyndryl, a US-based ICT provider, to ensure responsible development and governance of next-generation AI solutions (Gen AI).
Elevating Market Position through Innovations:
AI Cloud providers are actively advancing their product portfolios with innovative offerings to broaden their scope, enhance market positioning, and cultivate new capabilities. Microsoft, for example, is spearheading the development of proprietary AI chips, codenamed ‘Athena,’ designed to cater to various needs within Microsoft’s ecosystem while also addressing the chip requirements of OpenAI, anticipated to be available as early as 2024.
Offering Innovative and Tailor-Made Solutions:
AI Cloud providers are placing growing emphasis on customization and personalization, augmenting their offerings with supplementary services like Firewall/DDoS Protection and additional internet bandwidth tailored to meet individual user requirements. Moreover, they are implementing flexible pay-as-you-go payment models to stimulate market adoption.
Growing Preference of Hybrid Cloud for AI Deployment:
The hybrid cloud has emerged as the predominant infrastructure choice for driving AI initiatives, as it seamlessly integrates the advantages of on-premise infrastructure with the scalability and innovation potential offered by the cloud. According to recent data, approximately two out of every three enterprises leverage hybrid cloud environments to fuel their AI initiatives. Moreover, these enterprises have outlined plans to implement hybrid cloud solutions for up to 50% of their AI and machine learning (ML) projects by the year 2024.
Key Driving Forces of AI Cloud Growth:
Advancement in the Use of AI:
According to insights from Gartner’s Peer Community, a staggering 80% of business leaders have incorporated AI technology and solutions into their operations, reaping substantial business advantages like heightened productivity, enhanced product quality, and cost reduction. The primary drivers behind this adoption include a focus on improving product or service quality (78%), boosting staff productivity (68%), cutting operational expenses (37%), increasing sales (35%), and mitigating business risks (35%)
Adoption of Managed AI Services:
Enterprises are ramping up their digital transformation efforts by embracing both managed AI services and self-hosted AI solutions within cloud environments. According to Wiz Research, 70% of organizations are utilizing cloud-based managed AI services to enhance the efficiency of their business operations. Additionally, some organizations are tapping into self-hosted AI models like BERT and DistilBERT to train AI within their internal environments, further diversifying their AI strategies
Implementation of Gen AI or Predictive AI Solutions:
The adoption of Gen AI and Predictive AI technologies has experienced a notable surge, prompting cloud service providers to introduce both revamped and new solutions to cater to growing user demand. Enterprises are placing greater emphasis on Gen AI and predictive AI to drive efficiency, manage risks, and make informed decisions. Consequently, Gen AI adoption is projected to soar to 71% by 2025, according to a report published by KPMG in 2023.
Key Application Areas of AI Cloud:
Information Technology:
Customized Large Language Models (LLMs) tailored for the IT industry can revolutionize Natural Language Processing (NLP) applications, including chatbots and virtual assistants. These models can automate testing processes, comprehend complex code structures, and facilitate error debugging, thereby enhancing software development efficiency and quality.
Manufacturing:
Manufacturing companies can utilize LLMs to optimize supply chain management by predicting demand, identifying bottlenecks, and streamlining logistics. LLMs can also predict maintenance needs, reducing downtime and enhancing operational efficiency, while improving quality control processes to ensure product reliability and safety.
Retail:
LLMs empower retail enterprises to personalize customer interactions by analyzing consumer behavior and preferences, leading to tailored product recommendations and targeted marketing strategies. Additionally, these models optimize inventory management, analyze market trends, and forecast demand, enabling retailers to enhance operational efficiency and maximize profitability.
Healthcare:
In the healthcare sector, LLM models trained on extensive medical datasets facilitate medical documentation, research analysis, and the optimization of diagnostics and treatment strategies. By assisting in patient data analysis and clinical decision support, LLMs contribute to improved patient outcomes and healthcare delivery efficiency.
Banking, Financial Services, and Insurance (BFSI):
LLMs enable BFSI enterprises to make informed decisions by providing accurate market predictions and risk analysis. These models automate financial reports generation, compliance monitoring, and fraud detection, enhancing operational efficiency and regulatory compliance.
Legal Services:
Industry-specific LLMs support legal research, document analysis, and contract review tasks, improving efficiency and accuracy in legal processes. Trained on extensive legal databases, these models assist legal professionals in case preparation, contract drafting, and compliance assessment.
Logistics:
LLM-based bots streamline logistics operations by providing real-time shipping quotes, tracking shipments, and facilitating bookings. These bots optimize overall logistics processes, including route planning, inventory management, and last-mile delivery, leading to cost savings and improved customer satisfaction.
Education:
LLMs enhance educational experiences by generating customized learning materials, automating administrative tasks, and providing personalized feedback to students. Educational institutes utilize these models to develop adaptive learning platforms, analyze student performance data, and optimize curriculum design for better learning outcomes.
How AI Cloud Solves Business Problems:
Elevated Security & Threat Detection:
AI Cloud fortifies cloud security measures by swiftly identifying potential threats and vulnerabilities using machine learning algorithms and pattern recognition. Through proactive threat detection, enterprises can effectively thwart cyber attacks, safeguarding sensitive data and maintaining operational continuity.
Enhanced Productivity & Cost Efficiency:
Enterprises leverage real-time analytics provided by AI Cloud to optimize resource allocation, enhancing productivity while minimizing operational costs. By identifying inefficiencies and streamlining processes, organizations can reduce downtime and maximize resource utilization, thereby optimizing their cost-effectiveness.
Augmented Scalability and Flexibility:
AI Cloud empowers enterprises to scale their AI solutions seamlessly, adapting to evolving business requirements and optimizing resource allocation. With the flexibility to upscale or downscale AI resources as needed, organizations can efficiently respond to changes in demand and optimize resource utilization for enhanced efficiency.
Intelligent Process Automation:
AI Cloud enables enterprises to automate routine tasks and processes, driving efficiency gains and reducing reliance on manual intervention. By automating repetitive tasks, IT teams can focus on strategic initiatives, accelerating innovation and driving business growth.
Superior Customer Experience:
AI Cloud facilitates personalized customer interactions by analyzing data to anticipate needs, preferences, and behavior patterns. By offering tailored product recommendations, proactive support, and chat-based assistance, enterprises can enhance customer satisfaction and foster brand loyalty.
Enhanced Decision-Making:
Leveraging the data processing capabilities of AI Cloud, enterprises gain valuable insights from large volumes of data, enabling informed decision-making. By identifying trends, patterns, and correlations in data, organizations can make data-driven decisions that mitigate risks and capitalize on emerging opportunities.
Advanced Analytics:
AI Cloud offers robust analytics functionalities, encompassing predictive analytics, prescriptive analytics, and anomaly detection, empowering organizations to extract actionable insights from their data. Additionally, AI Cloud can analyze data from sensors and IoT devices to anticipate equipment failures preemptively, facilitating proactive maintenance and reducing downtime.
Language Translation and Natural Language Understanding:
AI Cloud provides language translation services and natural language understanding capabilities, enabling organizations to effectively communicate with customers and stakeholders in various languages. Additionally, it facilitates the comprehension of unstructured data, such as customer reviews and social media posts, aiding organizations in gaining valuable insights from diverse sources.
Potential Challenges that Come with AI Cloud:
Talent Acquisition and Skill Development:
The increasing adoption of AI Cloud solutions has led to a surge in demand for professionals with specialized skills. However, bridging the skill gap and providing ongoing training to keep up with evolving technologies remain significant challenges. Organizations struggle to recruit and retain talent proficient in AI technologies, hindering the effective implementation of AI Cloud initiatives.
Data Security and Privacy Management:
The AI algorithms employed in cloud environments process vast volumes of data, including sensitive information. This raises concerns regarding data security and privacy. Organizations must implement robust security measures to safeguard sensitive data against unauthorized access, breaches, and potential misuse.
Cybersecurity and Threat Mitigation:
As AI applications in the cloud become more sophisticated, they also become lucrative targets for cyber attackers. The complexity of AI algorithms and the interconnected nature of cloud environments increase the susceptibility to data breaches and cyberattacks. Ensuring robust cybersecurity measures and continuously monitoring for potential threats are essential to mitigate these risks.
Managing Compliance and Governance:
Integrating AI into cloud environments amplifies the complexity of regulatory and legal compliance requirements. Organizations must navigate multifaceted regulatory frameworks governing data protection, privacy, and ethical AI usage. Ensuring compliance with evolving regulations while maintaining operational efficiency poses significant challenges.
Integration Complexity and System Compatibility:
Integrating AI capabilities with existing cloud infrastructure presents numerous challenges, including compatibility issues, specialized skill requirements, and potential disruptions during integration. Organizations face complexities in harmonizing disparate systems and ensuring seamless interoperability while minimizing disruptions to ongoing operations.
Transparency and Accountability:
Transparency and explainability are crucial aspects of building trust in AI-driven systems. However, achieving transparency in AI algorithms poses challenges due to their inherent complexity. Organizations must ensure that users understand how AI makes decisions, fostering trust and accountability while addressing concerns related to bias and fairness.
Bias Mitigation and Ethical AI Practices:
AI algorithms deployed in the cloud can inadvertently perpetuate biases present in the training data, leading to unfair or discriminatory outcomes. Addressing bias in AI algorithms requires careful attention to data selection, algorithm design, and ongoing monitoring. Organizations must prioritize ethical considerations and implement measures to mitigate bias in AI-driven decision-making processes.
Optimal Resource Management:
Optimizing resource allocation and managing costs associated with AI Cloud deployments pose significant challenges for organizations. Balancing the need for computational resources with cost considerations requires careful planning and monitoring to avoid over-provisioning or underutilization of resources, optimizing performance while minimizing operational expenses.
Interoperability & Vendor Lock-In:
Integrating AI Cloud solutions from different vendors and ensuring interoperability across diverse platforms presents challenges related to vendor lock-in and data portability. Organizations must carefully evaluate vendor offerings, consider interoperability standards, and develop strategies to mitigate the risks associated with vendor lock-in, ensuring flexibility and agility in their AI Cloud deployments.
14 thoughts on “Harnessing the Power of AI in the Cloud (AI Cloud)”