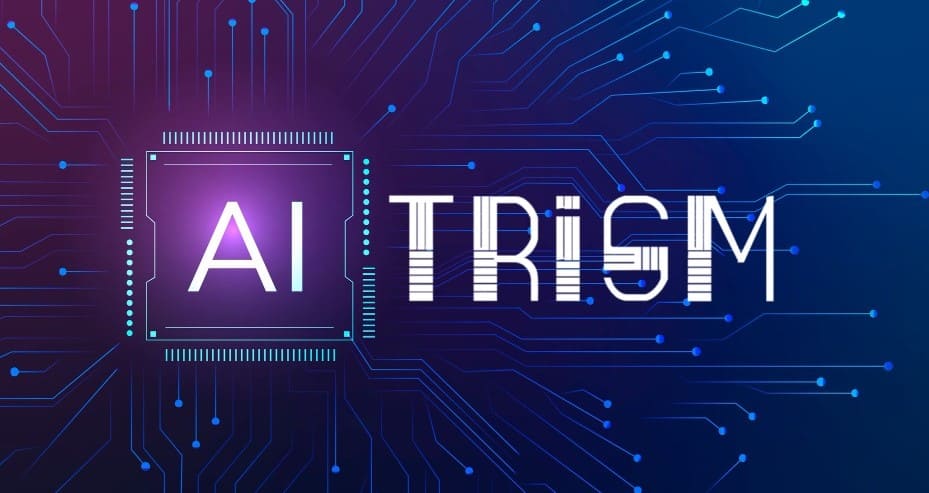
Overview:
The rapid evolution of artificial intelligence (AI) has brought about unprecedented opportunities and challenges across various sectors. As AI becomes increasingly integral to our daily lives and business operations, the need for robust frameworks to ensure its trustworthiness, risk management, and security has never been more critical. So, to understand what is AI TRiSM, we need to apprehend a framework designed to address the AI-related concerns and pave the way for the responsible and secure deployment of AI technologies. This article explores the futuristic tech landscape of AI TRiSM, highlighting the innovations and practices that will shape its development and implementation.
In the coming years, AI TRiSM is set to drive advancements that enhance the transparency, reliability, and security of AI systems. From advanced explainability tools and real-time risk management systems to comprehensive ethical frameworks and adaptive regulatory compliance mechanisms, AI TRiSM will play a pivotal role in ensuring that AI technologies are both beneficial and safe. As we integrate AI into various aspects of our lives, understanding the future of AI TRiSM becomes essential. This article examines key trends and innovations, emphasizing the challenges and opportunities that lie ahead, and how AI TRiSM will foster a landscape where AI can thrive responsibly and securely.
Contents:
- What is AI TRiSM
- Key Components of AI TRiSM
- Key Benefits of the AI TRiSM Framework
- Key Challenges in Implementing the AI TRiSM Framework
- Key Application Areas of AI TRiSM
- Future Outlook of AI TRiSM
- Summing Up
So, what is AI TRiSM:
AI TRiSM, or Artificial Intelligence Trust, Risk, and Security Management, is a structured approach ensuring the trustworthiness, fairness, reliability, robustness, efficacy, and data protection of AI models. It serves as a foundational framework for governing AI model practices, enabling organizations to proactively address risks associated with the deployment of AI technology.
The Key Components of AI TRiSM (AI Trust, Risk and Security Management):
1. Governance:
Provides structured policies and procedures to guide AI development and deployment, ensuring alignment with organizational goals and regulatory requirements. It establishes accountability and oversight mechanisms to manage AI initiatives effectively.
2. Risk Management:
Focuses on identifying, assessing, and mitigating potential risks associated with AI systems. This includes managing risks such as data privacy violations, algorithmic biases, and vulnerabilities in AI operations through proactive measures and continuous monitoring.
3. Compliance:
Ensures that AI systems adhere to established laws, regulations, and ethical guidelines. Regular audits and assessments verify compliance and promote transparency in AI operations to build trust with stakeholders.
4. Security:
Protects AI systems from external threats and unauthorized access through robust cybersecurity measures. This includes encryption, access controls, and threat detection systems to safeguard AI models, data, and infrastructure.
5. Ethics:
Addresses ethical concerns related to AI, such as fairness, transparency, and accountability. It ensures that AI systems operate without bias, respect user privacy, and uphold ethical standards in decision-making processes.
6. Explainability:
Enhances transparency by making AI systems and their processes understandable to human users. This mitigates the “black box” problem by providing insights into how AI models make decisions and handle data, fostering trust and facilitating effective human-AI collaboration.
7. ModelOps:
Involves lifecycle management of AI models, ensuring they are effectively integrated, monitored, and maintained within IT systems. This includes version control, performance monitoring, and scalability to optimize AI model deployment and usage.
8. Data Anomaly Detection:
Focuses on identifying and addressing anomalies in data that could impact AI system performance and reliability. It involves monitoring data quality, detecting deviations from expected patterns, and implementing corrective actions to maintain data integrity.
9. Adversarial Attack Resistance:
Ensures AI models can withstand and recover from adversarial attacks designed to manipulate or deceive them. This includes implementing defenses such as robust validation methods, adversarial training, and anomaly detection to enhance AI model resilience.
10. Data Protection:
Implements measures to safeguard sensitive data used by AI systems against unauthorized access, breaches, and misuse. This includes encryption, anonymization techniques, secure data storage, and access controls to protect data privacy and confidentiality.
Key Benefits of the AI TRiSM Framework:
1. Improved Trust and Transparency:
AI TRiSM fosters trust in AI systems by ensuring transparency in their decision-making processes. By making AI models more explainable and accountable, stakeholders can understand and trust the outcomes.
Example:
A financial institution using AI for loan approvals can use AI TRiSM to provide clear explanations for each decision, ensuring that customers and regulators understand how and why decisions are made.
2. Proactive Risk Mitigation:
The framework enables organizations to proactively identify and mitigate risks associated with AI models, such as algorithmic bias, data privacy breaches, and security vulnerabilities.
Example:
An e-commerce platform can use AI TRiSM to monitor and adjust algorithms that recommend products, ensuring they do not unfairly favor or disadvantage certain groups of users.
3. Enhanced Security and Data Protection:
AI TRiSM incorporates robust security measures, including encryption, access controls, and threat detection, to safeguard AI systems and the sensitive data they process.
Example:
A healthcare provider using AI for patient diagnosis can implement AI TRiSM to protect patient data from unauthorized access and ensure that AI models are secure against cyberattacks.
4. Regulatory Compliance:
By adhering to ethical guidelines and data privacy laws, AI TRiSM helps organizations ensure their AI deployments are compliant with relevant regulations.
Example:
A global company can use AI TRiSM to navigate and comply with varying data protection regulations across different regions, such as GDPR in Europe and CCPA in California.
5. Increased Adoption and User Acceptance:
According to Gartner, organizations that embrace AI transparency, trust, and security through AI TRiSM will see a 50% increase in AI model adoption and user acceptance.
Example:
A tech company developing a new AI-powered assistant can use AI TRiSM to ensure users feel confident in the assistant’s capabilities, leading to higher adoption rates.
6. Improved Business Outcomes:
The framework enables organizations to maximize the value of their AI investments by ensuring the reliability, fairness, and efficacy of AI models, leading to better business decisions and outcomes.
Example:
A retail chain using AI for inventory management can see improved accuracy in stock predictions and demand forecasting, resulting in reduced costs and enhanced customer satisfaction.
Key Challenges in Implementing the AI TRiSM Framework:
1. Complexity and Technical Expertise:
Implementing AI TRiSM requires deep technical expertise and a comprehensive understanding of AI technologies. Organizations may lack the necessary skills and knowledge, making it challenging to develop and maintain these frameworks.
Example:
A small business implementing AI for customer service might struggle to find and afford experts who can ensure AI TRiSM compliance.
2. Integration with Existing Systems:
Integrating AI TRiSM with existing IT infrastructure and workflows can be complex and resource-intensive. Ensuring compatibility and seamless operation with legacy systems poses a significant challenge.
Example:
A traditional manufacturing company adopting AI for predictive maintenance may face difficulties in integrating AI TRiSM into their existing production management systems.
3. Scalability:
Scaling AI TRiSM frameworks to handle large volumes of data and complex AI models across multiple applications and departments is a significant challenge.
Example:
A multinational corporation implementing AI TRiSM across different business units and geographical locations might face scalability issues, leading to inconsistent application and management of AI policies.
4. Bias and Fairness:
Addressing biases in AI models and ensuring fairness is a challenging aspect of AI TRiSM. Identifying and mitigating biases in data and algorithms requires continuous effort and sophisticated tools.
Example:
An AI system used in hiring processes must be regularly audited for biases to ensure fair treatment of all candidates, which requires ongoing monitoring and adjustment.
5. Resource Constraints:
Implementing and maintaining AI TRiSM frameworks can be resource-intensive, requiring significant investment in technology, personnel, and training.
Example:
Startups and small businesses may find it difficult to allocate sufficient resources to fully implement and sustain comprehensive AI TRiSM frameworks.
6. Cultural and Organizational Resistance:
Implementing AI TRiSM may face resistance from within the organization due to cultural and operational changes required. Gaining buy-in from all stakeholders and fostering a culture of trust and security can be difficult.
Example:
Employees in a traditional firm might resist new AI TRiSM protocols, perceiving them as an additional burden or fearing job displacement due to increased AI adoption.
Key Application Areas of AI TRiSM:
1. Manufacturing:
AI TRiSM optimizes production processes by monitoring equipment health for predictive maintenance and enhancing quality control.
Example:
A car manufacturer can use AI TRiSM to predict when machinery parts will fail, minimizing downtime and reducing maintenance costs while ensuring high-quality production standards.
2. Healthcare:
AI TRiSM aids in patient data analysis, disease diagnosis, and treatment recommendations, improving patient outcomes and reducing costs.
Example:
Hospitals can implement AI TRiSM to analyze patient records and detect early signs of diseases such as cancer, leading to timely and more effective treatments while safeguarding patient data privacy.
3. Logistics and Supply Chain:
AI TRiSM enhances supply chain visibility, demand forecasting, and route optimization, reducing operational costs and ensuring timely deliveries.
Example:
A logistics company can use AI TRiSM to optimize delivery routes based on real-time traffic data, reducing fuel consumption and ensuring faster delivery times, while maintaining the integrity of shipment data.
4. Finance:
AI TRiSM aids in fraud detection, risk assessment, and algorithmic trading, improving decision-making and security.
Example:
Banks can implement AI TRiSM to detect unusual transaction patterns indicative of fraud, ensuring robust security measures are in place to protect customer funds and personal information.
5. Retail and E-commerce:
AI TRiSM allows for personalized recommendations, customer experiences, and inventory management, enhancing the shopping experience and boosting sales.
Example:
An online retailer can use AI TRiSM to personalize product recommendations based on customer behavior, improving customer satisfaction and increasing sales, while also managing inventory more efficiently to meet demand.
6. Regulated Industries:
AI TRiSM is particularly beneficial in industries where compliance with ethical and legal requirements is mandatory, helping organizations demonstrate their commitment to responsible AI practices and mitigate potential risks.
Example:
Pharmaceutical companies can use AI TRiSM to ensure that AI-driven drug research complies with regulatory standards, thereby avoiding legal issues and ensuring safe, effective products reach the market.
7. Industries Handling Sensitive Data:
AI TRiSM ensures data protection, privacy, and integrity, maintaining customer trust and complying with regulations.
Example:
Insurance companies can use AI TRiSM to manage and protect sensitive customer information, ensuring compliance with data protection laws like GDPR, and maintaining customer trust by safeguarding personal data.
Future Outlook of AI TRiSM:
Market Landscape:
Gartner forecasts that by 2026, organizations that prioritize AI transparency, trust, and security will see a 50% rise in AI model adoption and user acceptance. As AI continues to take on a larger role in the economy, AI TRiSM is anticipated to become a leading-edge technology in the years ahead.
Technological Landscape:
1. Enhanced Explainability and Interpretability:
Advanced Model Explainability Tools: New tools and frameworks will emerge to make complex AI models, like deep learning, more interpretable. This will involve leveraging techniques like SHAP (Shapley Additive Explanations) and LIME (Local Interpretable Model-agnostic Explanations) on a broader scale.
User-Friendly Interfaces: Development of more intuitive interfaces that allow non-technical users to understand AI decisions and model behavior.
2. Proactive and Predictive Risk Management:
Real-Time Monitoring Systems: AI TRiSM will incorporate real-time monitoring to detect and address risks as they arise, using AI to predict potential failures or biases before they impact outcomes.
Automated Risk Mitigation: Automation of risk assessment and mitigation processes, allowing AI systems to self-correct and adapt to new threats dynamically.
3. Advanced Security Measures:
AI-Driven Cybersecurity: Use of AI to bolster cybersecurity, identifying and responding to threats faster than traditional methods.
Quantum-Resistant Encryption: Development and implementation of quantum-resistant cryptographic techniques to protect data as quantum computing becomes more prevalent.
4. Collaborative Human-AI Interaction:
Enhanced Feedback Loops: Development of advanced systems to facilitate better human-AI collaboration, incorporating human feedback into AI learning processes more effectively.
Interactive AI Systems: Creation of more interactive AI systems that can explain their actions and decisions in real-time to users, fostering greater trust and collaboration.
5. Data Privacy and Protection Innovations:
Federated Learning: Adoption of federated learning techniques to train AI models across decentralized data sources without compromising data privacy.
Differential Privacy: Implementation of differential privacy methods to ensure individual data points cannot be reverse-engineered from aggregated AI models.
6. Scalable and Flexible AI TRiSM Solutions:
Modular Frameworks: Development of modular AI TRiSM frameworks that can be easily scaled and adapted to different organizational needs and sizes.
Cloud-Based AI TRiSM Services: Proliferation of cloud-based AI TRiSM services offering scalable, flexible, and cost-effective solutions for businesses of all sizes.
7. Integration with Emerging Technologies:
IoT and AI Integration: Integration of AI TRiSM with the Internet of Things (IoT) to manage the risks and security of interconnected devices.
Edge Computing: Use of edge computing to enhance the performance and security of AI systems by processing data closer to the source.
Summing Up:
In conclusion, the future of AI TRiSM (Artificial Intelligence Trust, Risk, and Security Management) is both promising and essential for the continued integration of AI into our daily lives and business operations. As AI technologies become more pervasive, the need for frameworks that ensure their trustworthiness, mitigate risks, and enhance security becomes increasingly critical. By embracing advanced explainability tools, real-time risk management systems, ethical frameworks, and adaptive compliance mechanisms, AI TRiSM will play a crucial role in fostering a safe and responsible AI landscape.
The evolution of AI TRiSM will be marked by continuous innovation and the adoption of emerging technologies, ensuring that AI systems remain transparent, reliable, and secure. Organizations that prioritize AI TRiSM will be better positioned to leverage the full potential of AI, gaining user trust and achieving greater adoption rates. As we move forward, the commitment to robust AI TRiSM practices will be instrumental in navigating the complexities of AI deployment, ultimately leading to a future where AI technologies can thrive ethically and securely, driving positive outcomes across various sectors.
Image Courtesy: FreePik